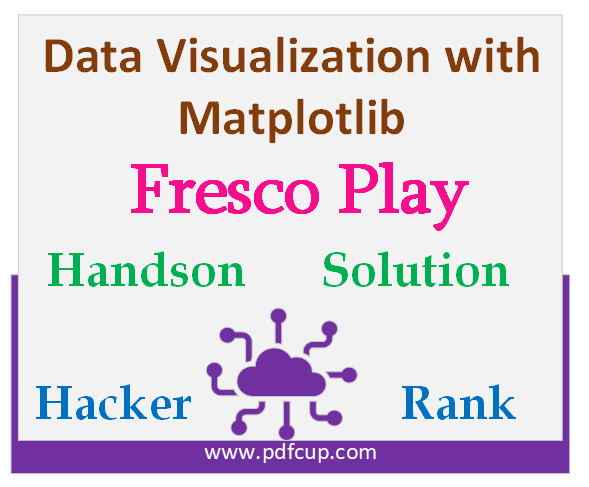
Data Visualization with Matplotlib Fresco Play Hands on:-
LAB 1: Matplotlib-1 My First Plot.
Solution 1: Matplotlib-1 My First Plot.
import numpy as np import matplotlib.pyplot as plt def test_the_plot(): # Write your functionality below # Create a figure of size 12 inches in width, and 6 inches in height. Name it as fig. fig = plt.figure(figsize=(12, 6)) # Create an axis, associated with figure fig, using add_subplot. Name it as ax. ax = fig.add_subplot(111) # Create a list t with values [7, 14, 21, 28, 35] t = [7, 14, 21, 28, 35] # Create a list d with values [i*6 for i in t] d = [i*6 for i in t] x = t y = d # Draw a line, by plotting t values on X-Axis and d values on Y-Axis. Use the plot function. Label the line as d = 6t. plt.plot(x, y, label='d = 6t') # Label X-Axis as time (second). Label Y-Axis as distance(meters). # Set Title as 'Time vs Distance Covered' # Limit data points on X-Axis from 0 to 40. # Limit data points on Y-Axis from 0 to 220. ax.set(title='Time vs Distance Covered', xlabel='time (seconds)', ylabel="distance (meters)", xlim=(0, 40), ylim=(0, 220)) plt.legend() return fig # Refer '__main__' method code which is given below if required.
if __name__ == '__main__':
# Starting from here: # Note: Do Not modify the below code if __name__ == '__main__': import sys import subprocess subprocess.check_call( [sys.executable, "-m", "pip", "install", 'test_plot1-0.2-py3-none-any.whl'], stdout=subprocess.DEVNULL) from test_plot1 import matplotlib1 usr_fig = test_the_plot() matplotlib1.save_answer(usr_fig)
LAB 2: Welcome to Matplotlib 2 Scatter Plots.
Solution 2: Welcome to Matplotlib 2 Scatter Plots.
import numpy as np import matplotlib.pyplot as plt def sine_wave_plot(): # Write your functionality below # Create a figure of size 13 inches in width, and 4 inches in height. Name it as fig. fig = plt.figure(figsize=(13, 4)) # Create an axis, associated with figure fig, using add_subplot. Name it as ax. ax = fig.add_subplot(111) # Create a numpy array arr_t with 250 values between 0.0 and 3.0. USe the 'linespace' method to generate 250 values. arr_t = np.linspace(0.0, 3.0, 250) # Create a numpy array arr_v such that arr_v= np.sin(2.5*np.pi*arr_t). arr_v = np.sin(2.5*np.pi*arr_t) # Pass arr_t and arr_v as variable to plot function and draw a red line passing through the selected 250 points. # Label the line as sin(arr_t). x = arr_t y = arr_v plt.plot(x, y, label='sin(arr_t)', color='red') # Label X_Axis as Time(seconds), Label Y-Axis as Voltage(mv). # Set Title as Sine Wave. # Limit data on X-Axis from 0 to 2, Limit data on Y-Axis from -1 to 1. ax.set(title='Sine Wave', xlabel='Time (seconds)', ylabel="Voltage (mv)", xlim=(0,2), ylim=(-1, 1)) # Mark major ticks on X_Axis at 0, 0.2, 0.4, 0.6, 0.8, 1.0, 1.2, 1.4, 1.6, 1.8, 2.0. plt.xticks([0, 0.2, 0.4, 0.6, 0.8, 1.0, 1.2, 1.4, 1.6, 1.8, 2.0]) # Mark major ticks on Y-Axis at -1,0, and 1. plt.yticks([-1, 0, 1]) # Add a grid, whose linestyle is dashdot. plt.grid(linestyle='--', which='major') plt.legend() return fig def multi_curve_plot(): # Write your functionality below # Create a figure of size 13 inches in width, and 4 inches in height. Name it as fig. fig = plt.figure(figsize=(13, 4)) # Create an axis, associated with figure fig, using add_subplot. Name it as ax. ax = fig.add_subplot(111) # Create a numpy array arr_x with 25 values between 0.0 and 7.0. USe the 'linespace' method to generate 25 values. arr_x = np.linspace(0, 7, 25) # Create three numpy arrays arr_y1, arr_y2 and arr_y3, using the expression arr_y1 = arr_x, arr_y2 = arr_x**2 and arr_y3 = arr_x**3. arr_y1 = arr_x arr_y2 = arr_x**2 arr_y3 = arr_x**3 # Draw a green colored line passing through arr_x and arr_y1, using the plot function. # Mark the 25 data points on the lines as upward pointed triangles. Label the as y = arr_x. plt.plot(arr_x, arr_y1, label='y = arr_x', color='green', marker="^") # Draw a blue colored line passing through arr_x and arr_y2, using the plot function. # Mark the 25 data points on the lines as Squares. Label the as y = arr_x**2. plt.plot(arr_x, arr_y2, label='y = arr_x**2', color='blue', marker='s') # Draw a red colored line passing through arr_x and arr_y3, using the plot function. # Mark the 25 data points on the lines as Circles. Label the as y = arr_x**3. plt.plot(arr_x, arr_y3, label='y = arr_x**3', color='red', marker='o') # Label X-Axis as arr_x. Label Y_Axis as f(arr_x) # Set the Title as Linear, Quadratic, & Cubic Equations. ax.set(title='Linear, Quadratic, & Cubic Equations', xlabel='arr_x', ylabel="f(arr_x)") plt.legend() return fig def scatter_plot(): # Write your functionality below. # Create a figure of size 13 inches in width, and 4 inches in height. Name it as fig. fig = plt.figure(figsize=(13, 4)) # Create an axis, associated with figure fig, using add_subplot. Name it as ax. ax = fig.add_subplot(111) # Consider the list ca_sales= [40, 65, 70, 40, 55, 60, 75, 60, 80, 95, 96, 105]. It represents the number of cars sold by a Company 'X' in each month of 2021, starting from January, 2021. car_sales = [40, 65, 70, 40, 55, 60, 75, 60, 80, 95, 96, 105] # Create a list of months contaning numbers from 1 to 12 months = [1, 2, 3, 4, 5, 6, 7, 8, 9, 10, 11, 12] # Draw a scatter plot with variables months and car_sales as arguments. Mark the data points in green color. Use the scatter function for plotting. Label the points as car sales. ax.scatter(months, car_sales, color='green', marker='o') # Limit data on X-Axis from 0 to 13. Limit data on Y-Axis from 10 to 110. # Mark ticks on X-Axis at 1,3,5,7,9, and 11. # Label the X-Axis ticks as January, March, May, July, Sepetember, and November respectively. # Set Title as "Cars Sold by Company 'Z' in 2021". ax.set(title="Cars Sold by Company 'Z' in 2021", xlabel='Months', ylabel='No. of Cars Sold', xlim=(0, 13), ylim=(10, 110)) plt.xticks([1, 3, 5, 7, 9, 11], ['January', 'March', 'May', 'July', 'September', 'November']) plt.legend() return fig # Refer '__main__' method code which is given below if required.
if __name__ == '__main__':
# Starting from here: # Note: Do Not modify the below code if __name__ == '__main__': import sys import subprocess subprocess.check_call( [sys.executable, "-m", "pip", "install", 'test_plot2-0.1-py3-none-any.whl'], stdout=subprocess.DEVNULL) from test_plot2 import matplotlib2 usr_fig1 = sine_wave_plot() usr_fig2 = multi_curve_plot() usr_fig3 = scatter_plot() matplotlib2.save_answer(usr_fig1, usr_fig2, usr_fig3)
LAB 3 Welcome to Matplotlib -3 - Bar Plots.
Solution 3: Welcome to Matplotlib -3 - Bar Plots.
import numpy as np import matplotlib.pyplot as plt def barplot_of_iris_sepal_length(): # Write your functionality below # Create a figure of size 9 inches in width, and 7 inches in height. Name it as fig. fig = plt.figure(figsize=(9, 7)) # Create an axis, associated with figure fig, using add_subplot. Name it as ax. ax = fig.add_subplot(111) # Define a list species, with elements ['setosa', 'versicolor', 'virginiica'] species = ['setosa', 'versicolor', 'virginica'] # Define a list index, with values [0.4, 1.4, 2.4]. index = [0.4, 1.4, 2.4] # Define another list sepal_lenwith values [6.01, 6.94, 7.69]. These values represent the mean sepal length of iris flowers belonging to three species. sepal_len = [6.01, 6.94, 7.69] # Draw a bar plot using the bar function, such that the height of each vertical bar display the sepal length of a species label it as Sepal Length. # Use index and sepal_len as variables. Set bar width as 0.4, color as blue, and border color of the bar as red. ax.bar(index, sepal_len, color='blue', width=0.4, edgecolor='red', label='Sepal Length') # Label X-Axis as 'Species', Label Y-Axis as 'Sepal Length (cm)'. # Set Title as 'Mean Sepal Length of Iris Species' # Limit X-Axis from 0 to 3, Limit Y-Axis from 0 to 9. ax.set(title='Mean Sepal Length of Iris Species', xlabel='Species', ylabel='Sepal Length (cm)', xlim=(0, 3), ylim=(0, 9)) # Set ticks on X-Axis at 0.4, 1.4, and 2.4. ax.set_xticks(index) # Set X_Axis tick labels to ['setosa', 'versicolor', 'virginica']. ax.set_xticklabels(species) ax.legend() return fig def barplot_of_iris_measurements(): # Write your functionality below # Create a figure of size 9 inches in width, and 7 inches in height. Name it as fig. fig = plt.figure(figsize=(9, 7)) # Create an axis, associated with figure fig, using add_subplot. Name it as ax. ax = fig.add_subplot(111) # Define the Following lists: sepal_len = [6.01, 6.94, 7.59] sepal_wd = [4.42, 3.77, 3.97] petal_len = [2.46, 5.26, 6.55] petal_wd = [1.24, 2.33, 3.03] species = ['setosa', 'versicolor', 'virginica'] species_index1 = [0.7, 1.7, 2.7] species_index2 = [0.9, 1.9, 2.9] species_index3 = [1.1, 2.1, 3.1] species_index4 = [1.3, 2.3, 3.3] # Draw vertical bars showing the mean sepal length of a species. # Set the color of the bars to m, boundary line color to grey, width of bars as 0.2, and label it as Sepal Length. # Use bar with species_index1 and sepal_len. ax.bar(species_index1, sepal_len, width=0.2, color='m', edgecolor='grey', label='Sepal Length') # Draw vertical bars showing the mean sepal length of a species. # Set the color of the bars to y, boundary line color to grey, width of bars as 0.2, and label it as Sepal Width. # Use bar with species_index2 and sepal_wd. ax.bar(species_index2, sepal_wd, width=0.2, color='y', edgecolor='grey', label='Sepal Width') # Draw vertical bars showing the mean sepal length of a species. # Set the color of the bars to c, boundary line color to grey, width of bars as 0.2, and label it as Petal Length. # Use bar with species_index3 and petal_len. ax.bar(species_index3, petal_len, width=0.2, color='c', edgecolor='grey', label='Petal Length') # Draw vertical bars showing the mean sepal length of a species. # Set the color of the bars to orange, boundary line color to grey, width of bars as 0.2, and label it as Petal Width. # Use bar with species_index4 and petal_wd. ax.bar(species_index4, petal_wd, width=0.2, color='orange', edgecolor='grey', label='Petal Width') # Label X-Axis as 'Species', Label Y-Axis as 'Iris Measurements (cm)'. # Set Title as 'Mean Measurements of Ires Species' # Limit X-Axis from 0.5 to 3.5, Limit Y-Axis from 0 to 10. ax.set(title='Mean Measurements of Ires Species', xlabel='Species', ylabel='Iris Measurements (cm)', xlim=(0.5, 3.5), ylim=(0, 10)) # Mark major tick on X-Axis at 1.0,2.0,3.0 ax.set_xticks([1.0, 2.0, 3.0]) # Lable th emajor ticks on X-Axis as setosa, versicolor and virginica respectively. ax.set_xticklabels(species) ax.legend() return fig def hbarplot_of_iris_petal_length(): # Write your functionality below fig = plt.figure(figsize=(15, 5)) ax = fig.add_subplot(111) species = ['setosa', 'versicolor', 'virginica'] index = [0.1, 1.1, 2.1] petal_len = [2.67, 5.49, 6.37] ax.set(title='Mean Petal Length of Iris Species', xlabel='Petal Length (cm)', ylabel='Species') ax.barh(index, petal_len, height=0.4, color='m', edgecolor='c') ax.set_yticks([0.10, 1.10, 2.10]) ax.set_yticklabels(species) ax.legend() return fig # Refer '__main__' method code which is given below if required.
if __name__ == '__main__':
# Starting from here: # Note: Do Not modify the below code if __name__ == '__main__': import sys import subprocess subprocess.check_call( [sys.executable, "-m", "pip", "install", 'test_plot3-0.1-py3-none-any.whl'], stdout=subprocess.DEVNULL) from test_plot3 import matplotlib3 usr_fig1 = barplot_of_iris_sepal_length() usr_fig2 = barplot_of_iris_measurements() usr_fig3 = hbarplot_of_iris_petal_length() matplotlib3.save_answer(usr_fig1, usr_fig2, usr_fig3)
LAB 4: Welcome to Matplotlib -4 - Histograms and Box Plots.
Solution 4: Welcome to Matplotlib -4 - Histograms and Box Plots.
import numpy as np import matplotlib.pyplot as plt def hist_of_a_sample_normal_distribution(): # Write your functionality below # Create a figure of size 9 inches in width, and 7 inches in height. Name it as fig. fig = plt.figure(figsize=(9, 7)) # Create an axis, associated with figure fig, using add_subploat. Name it as ax. ax = fig.add_subplot(111) # Set random see to 100 using the expression np.random.seed(100) np.random.seed(100) # Create a normal distribution dist_arr of 1000 values, with mean 35 and standard deviation 3.0. Use the hist function. dist_arr = 35 + 3.0*np.random.randn(1000) # Label X-Axis as dist_arr, Label Y-Axis as 'Bin Count'. # Set title as Histogram of a Single Dataset. ax.set(title="Histogram of a Single Dataset", ylabel='Bin Count', xlabel='dist_arr') ax.hist(dist_arr, bins=35) return fig def boxplot_of_four_normal_distribution(): # Write your functionality below. # Create a figure of size 9 inches in width, and 7 inches in height. Name it as fig. fig = plt.figure(figsize=(9, 7)) # Create an axis, associated with figure fig, using add_subploat. Name it as ax. ax = fig.add_subplot(111) # Set random see to 100 using the expression np.random.seed(100) np.random.seed(100) # Create a normal distribution arr_1 of 2000 values, with mean 35 and standard deviation 6.0. Use np.random.randn. arr_1 = 35 + 6.0*np.random.randn(2000) # Create a normal distribution arr_2 of 2000 values, with mean 25 and standard deviation 4.0. Use np.random.randn. arr_2 = 25 + 4.0*np.random.randn(2000) # Create a normal distribution arr_3 of 2000 values, with mean 45 and standard deviation 8.0. Use np.random.randn. arr_3 = 45 + 8.0*np.random.randn(2000) # Create a normal distribution arr_4 of 2000 values, with mean 55 and standard deviation 10.0. Use np.random.randn. arr_4 = 55 + 10.0*np.random.randn(2000) # Create a list labels with elements ['arr_1', 'arr_2', 'arr_3', 'arr_4'] labels = ['arr_1', 'arr_2', 'arr_3', 'arr_4'] # Draw a Boxplot arr_1, arr_2, arr_3, arr_4 with notches and label it using the labels list. USe the boxplot function. # Choose 'o' symbol for outlier, and fill color inside boxes by setting patch_artist argument to True. norml_dist_list = [arr_1, arr_2, arr_3, arr_4] ax.boxplot(norml_dist_list, labels=labels, notch=True, sym='o', patch_artist=True) # Label X_Axes as 'Dataset', Label Y-Axes as 'Value'. Set Title as "Box Plot of Multiple Dataset". ax.set(title="Box Plot of Multiple Dataset", ylabel='Value', xlabel='Dataset') return fig # Refer '__main__' method code which is given below if required.
if __name__ == '__main__':
# Starting from here: # Note: Do Not modify the below code if __name__ == '__main__': import sys import subprocess subprocess.check_call( [sys.executable, "-m", "pip", "install", 'test_plot4-0.1-py3-none-any.whl'], stdout=subprocess.DEVNULL) from test_plot4 import matplotlib4 usr_fig1 = hist_of_a_sample_normal_distribution() usr_fig2 = boxplot_of_four_normal_distribution() matplotlib4.save_answer(usr_fig1, usr_fig2)
LAB 5: Welcome to Matplotlib -5 - Applying Styles.
Solution 5: Welcome to Matplotlib -5 - Applying Styles.
# import numpy as np import matplotlib.pyplot as plt # Task 1: def generate_plot_with_style1(): # Write your functionality below # Create a function barplot with 'ggplot' style. Use 'with' to apply the style to the code generating the barplot. with plt.style.context(['ggplot']): # Create a figure of size 9 inches in width, and 7 inches in height. Name it as fig. fig = plt.figure(figsize=(9, 7)) # Create an axis, associated with figure fig, using add_subplot. Name it as ax. ax = fig.add_subplot(111) # Define the Following lists: sepal_len = [6.01, 6.94, 7.59] sepal_wd = [4.42, 3.77, 3.97] petal_len = [2.46, 5.26, 6.55] petal_wd = [1.24, 2.33, 3.03] species = ['setosa', 'versicolor', 'virginica'] species_index1 = [0.8, 1.8, 2.8] species_index2 = [1.0, 2.0, 3.0] species_index3 = [1.2, 2.2, 3.2] species_index4 = [1.4, 2.4, 3.4] # Draw vertical bars showing mean sepal length of a species. Set width of the bars as 0.2, and label it as Sepal Length. # Use bar with species_index1 and sepal_len. ax.bar(species_index1, sepal_len, width=0.2, label='Sepal Length') # Draw vertical bars showing mean sepal length of a species. Set width of the bars as 0.2, and label it as Sepal Width. # Use bar with species_index2 and sepal_wd. ax.bar(species_index2, sepal_wd, width=0.2, label='Sepal Width') # Draw vertical bars showing mean sepal length of a species. Set width of the bars as 0.2, and label it as Petal Length. # Use bar with species_index3 and petal_len. ax.bar(species_index3, petal_len, width=0.2, label='Petal Length') # Draw vertical bars showing mean sepal length of a species. Set width of the bars as 0.2, and label it as Petal Width. # Use bar with species_index4 and petal_wd. ax.bar(species_index4, petal_wd, width=0.2, label='Petal Width') # Label X-Axis as Species. # Label Y-Axis as Iris Measurements (cm) # Set Title as 'Mean Measurements of Ires Species' # Limit X-Axis from 0.5 to 3.7 # Limit Y-Axis from 0 to 10. ax.set(title='Mean Measurements of Ires Species', xlabel='Species', ylabel='Iris Measurements (cm)', xlim=(0.5, 3.7), ylim=(0, 10)) # Mark major ticks on X-Axis at 1.0,2.0, and3.0. # Label the major ticks on X-Axis as setosa, versicolor, and virginica respectively. ax.set_xticks([1.0, 2.0, 3.0]) ax.set_xticklabels(species) return fig # Task 2: def generate_plot_with_style2(): # Write your functionality below # Regenerate the barplot defined in 'generate_plot_with_style1' using 'seaborn-colorblind' style. # Use 'with' for applying the style. with plt.style.context(['seaborn-colorblind']): fig = plt.figure(figsize=(9, 7)) ax = fig.add_subplot(111) sepal_len = [6.01, 6.94, 7.59] sepal_wd = [4.42, 3.77, 3.97] petal_len = [2.46, 5.26, 6.55] petal_wd = [1.24, 2.33, 3.03] species = ['setosa', 'versicolor', 'virginica'] species_index1 = [0.8, 1.8, 2.8] species_index2 = [1.0, 2.0, 3.0] species_index3 = [1.2, 2.2, 3.2] species_index4 = [1.4, 2.4, 3.4] # Limit X-Axis from 0.5 to 3.5 ax.set(title='Mean Measurements of Ires Species', xlabel='Species', ylabel='Iris Measurements (cm)', xlim=(0.5, 3.5), ylim=(0, 10)) ax.bar(species_index1, sepal_len, width=0.2, label='Sepal Length') ax.bar(species_index2, sepal_wd, width=0.2, label='Sepal Width') ax.bar(species_index3, petal_len, width=0.2, label='Petal Length') ax.bar(species_index4, petal_wd, width=0.2, label='Petal Width') ax.set_xticks([1.0, 2.0, 3.0]) ax.set_xticklabels(species) return fig # Task 3: def generate_plot_with_style3(): # Write your functionality below # Regenerate the barplot defined in 'generate_plot_with_style1' using 'grayscale' style. # Use 'with' for applying the style. with plt.style.context(['grayscale']): fig = plt.figure(figsize=(9, 7)) ax = fig.add_subplot(111) sepal_len = [6.01, 6.94, 7.59] sepal_wd = [4.42, 3.77, 3.97] petal_len = [2.46, 5.26, 6.55] petal_wd = [1.24, 2.33, 3.03] species = ['setosa', 'versicolor', 'virginica'] species_index1 = [0.8, 1.8, 2.8] species_index2 = [1.0, 2.0, 3.0] species_index3 = [1.2, 2.2, 3.2] species_index4 = [1.4, 2.4, 3.4] # Limit X-Axis from 0.5 to 3.5 ax.set(title='Mean Measurements of Ires Species', xlabel='Species', ylabel='Iris Measurements (cm)', xlim=(0.5, 3.5), ylim=(0, 10)) ax.bar(species_index1, sepal_len, width=0.2, label='Sepal Length') ax.bar(species_index2, sepal_wd, width=0.2, label='Sepal Width') ax.bar(species_index3, petal_len, width=0.2, label='Petal Length') ax.bar(species_index4, petal_wd, width=0.2, label='Petal Width') ax.set_xticks([1.0, 2.0, 3.0]) ax.set_xticklabels(species) return fig # Refer '__main__' method code which is given below if required.
if __name__ == '__main__':
# Starting from here: # Note: Do Not modify the below code if __name__ == '__main__': import sys import subprocess subprocess.check_call( [sys.executable, "-m", "pip", "install", 'test_plot5-0.1-py3-none-any.whl'], stdout=subprocess.DEVNULL) from test_plot5 import matplotlib5 usr_fig1 = generate_plot_with_style1() usr_fig2 = generate_plot_with_style2() usr_fig3 = generate_plot_with_style3() matplotlib5.save_answer(usr_fig1, usr_fig2, usr_fig3)
LAB 6: Welcome to Matplotlib -6 - Multiple Plots.
Solution 6: Welcome to Matplotlib -6 - Multiple Plots.
# import numpy as np import matplotlib.pyplot as plt import matplotlib.gridspec as gridspec # Task 1: def generate_figure1(): # Write your functionality below # Define a numpy array 'x' with expression 'np.arrange(0.0,10.0, 0.01)' x = np.arange(0.0, 10.0, 0.01) # Define another numpy array 'arr_s1' with expression 'np.sin(3*np.pi*x)' arr_s1 = np.sin(3*np.pi*x) # Define another numpy array 'arr_s2' with expression 'np.sin(6*np.pi*x)' arr_s2 = np.sin(6*np.pi*x) # Create figure of size 12 inches in width, and 7 inches in hight. Name it as fig. fig = plt.figure(figsize=(12, 7)) # Create an axes, using plt.subplot function. Name it as axes1. # The subplot must point to the first virtual grid created by 2 rows and 1 columns. # Set 'title' argument to 'Sin(3*pi*x)'. axes1 = plt.subplot(2, 1, 1, title='Sin(3*pi*x)') # Draw a line plot of 'x' and 'arr_s1' using the 'plot' function on 'axes1'. axes1.plot(x, arr_s1) # Create another axes, using plt.subplot function. Name it as axes2. # The subplot must point to the second virtual grid created by 2 rows and 1 columns. # Set 'title' argument to 'Sin(6*pi*x)'. Set 'sharex' argument to 'axes1' and 'sharey' to 'axes1'. axes2 = plt.subplot(2, 1, 2, title='Sin(6*pi*x)', sharex=axes1, sharey=axes1) # Draw a line plot of 'x' and 'arr_s2' using the 'plot' function on 'axes2'. axes2.plot(x, arr_s2) return fig # Task 2: def generate_figure2(): # Write your functionality below # Set random seed to 1500 using the expression 'np.random.seed(1500)'. np.random.seed(1500) # Define a numpy array 'x' with expression 'np.random.rand(10)' x = np.random.rand(10) # Define another numpy array 'y' with expression 'np.random.rand(10)' y = np.random.rand(10) # Define one more numpy array 'z' with expression 'np.sqrt(x**2 + y*2)' z = np.sqrt(x**2 + y**2) # Create a figure of size 9 inches in width, and 7 inches in height. Name it as fig. fig = plt.figure(figsize=(9, 7)) # Create an axes, using plt.subplot function. Name it as axes1. # The subplot must point to the first virtual grid created by 2 rows and 2 columns. # Set 'title' argument to 'Scatter plot with Diamond Markers'. axes1 = plt.subplot(2, 2, 1, title='Scatter plot with Diamond Markers') # Draw a scatter plot of 'x' and 'y' using 'scatter' function on 'axes1'. # Set arguement 's' to 80, 'c' to z and 'marker' to 'd'. axes1.scatter(x, y, s=80, c=z, marker="d") # Add ticks on X-Axis at 0.0,0.5,1.0,1.5 and ticks on Y-Axis at -0.2, 0.2, 0.6, 1.0 respectively. axes1.set_xticks([0.0, 0.5, 1.0, 1.5]) axes1.set_yticks([-0.2, 0.2, 0.6, 1.0]) # Create an axes, using plt.subplot function. Name it as axes2. # The subplot must point to the Second virtual grid created by 2 rows and 2 columns. # Set 'title' argument to 'Scatter plot with Circle Markers'. axes2 = plt.subplot(2, 2, 2, title='Scatter plot with Circle Markers') # Draw a scatter plot of 'x' and 'y' using 'scatter' function on 'axes2'. # Set arguement 's' to 80, 'c' to z and 'marker' to 'o'. axes2.scatter(x, y, s=80, c=z, marker="o") # Add ticks on X-Axis at 0.0,0.5,1.0,1.5 and ticks on Y-Axis at -0.2, 0.2, 0.6, 1.0 respectively. axes2.set_xticks([0.0, 0.5, 1.0, 1.5]) axes2.set_yticks([-0.2, 0.2, 0.6, 1.0]) # Create an axes, using plt.subplot function. Name it as axes3. # The subplot must point to the Third virtual grid created by 2 rows and 2 columns. # Set 'title' argument to 'Scatter plot with Plus Markers'. axes3 = plt.subplot(2, 2, 3, title='Scatter plot with Plus Markers') # Draw a scatter plot of 'x' and 'y' using 'scatter' function on 'axes3'. # Set arguement 's' to 80, 'c' to z and 'marker' to '+'. axes3.scatter(x, y, s=80, c=z, marker="+") # Add ticks on X-Axis at 0.0,0.5,1.0,1.5 and ticks on Y-Axis at -0.2, 0.2, 0.6, 1.0 respectively. axes3.set_xticks([0.0, 0.5, 1.0, 1.5]) axes3.set_yticks([-0.2, 0.2, 0.6, 1.0]) # Create an axes, using plt.subplot function. Name it as axes4. # The subplot must point to the Fourth virtual grid created by 2 rows and 2 columns. # Set 'title' argument to 'Scatter plot with Upper Triangle Markers'. axes4 = plt.subplot(2, 2, 4, title='Scatter plot with Upper Triangle Markers') # Draw a scatter plot of 'x' and 'y' using 'scatter' function on 'axes4'. # Set arguement 's' to 80, 'c' to z and 'marker' to '^'. axes4.scatter(x, y, s=80, c=z, marker="^") # Add ticks on X-Axis at 0.0,0.5,1.0,1.5 and ticks on Y-Axis at -0.2, 0.2, 0.6, 1.0 respectively. axes4.set_xticks([0.0, 0.5, 1.0, 1.5]) axes4.set_yticks([-0.2, 0.2, 0.6, 1.0]) plt.tight_layout() return fig # Task 3: def generate_figure3(): # Write your functionality below # Define a numpy array X with expression 'np.arrange(1,301,3)' x = np.arange(1, 301, 3) # Define another numpy array 'y1' with expression 'y1= x' y1 = x # Define another numpy array 'y2' with expression 'y2 = x**2' y2 = x**2 # Define another numpy array 'y3' with expression 'y3= x**3' y3 = x**3 # Create a figure of size 9 inhes in width, and 7 inches in height. Name it as fig. fig = plt.figure(figsize=(9, 7)) # Define a grid 'gr' of 2 rows and 2 coloumns, using 'GridSpec' function. Ensure that 'matplotlib.gridspec' is imported, before defining the grid. gr = gridspec.GridSpec(2, 2) # Create an axes, using plt.subplot function. Name it as axes1. # The subplot must span the 1st row and 1st column of the defined grid 'gr'. # Set 'title' argument to 'y=x'. axes1 = plt.subplot(gr[0, 0], title='y = x') # Draw a line plot of 'x' and 'y1' using 'plot' function on 'axes1'. axes1.plot(x, y1) # Create an axes, using plt.subplot function. Name it as axes2. # The subplot must span the 2nd row and 1st column of the defined grid 'gr'. # Set 'title' argument to 'y=x**2'. axes2 = plt.subplot(gr[1, 0], title='y = x**2') # Draw a line plot of 'x' and 'y2' using 'plot' function on 'axes2'. axes2.plot(x, y2) # Create an axes, using plt.subplot function. Name it as axes3. # The subplot must span all rows and 2nd column of the defined grid 'gr'. # Set 'title' argument to 'y=x**3'. axes3 = plt.subplot(gr[:, 1], title='y = x**3') # Draw a line plot of 'x' and 'y3' using 'plot' function on 'axes3'. axes3.plot(x, y3) plt.tight_layout() return fig # Refer '__main__' method code which is given below if required.
if __name__ == '__main__':
# Starting from here: # Note: Do Not modify the below code if __name__ == '__main__': import sys import subprocess subprocess.check_call( [sys.executable, "-m", "pip", "install", 'test_plot6-0.2-py3-none-any.whl'], stdout=subprocess.DEVNULL) from test_plot6 import matplotlib6 usr_fig1 = generate_figure1() usr_fig2 = generate_figure2() usr_fig3 = generate_figure3() matplotlib6.save_answer(usr_fig1, usr_fig2, usr_fig3)