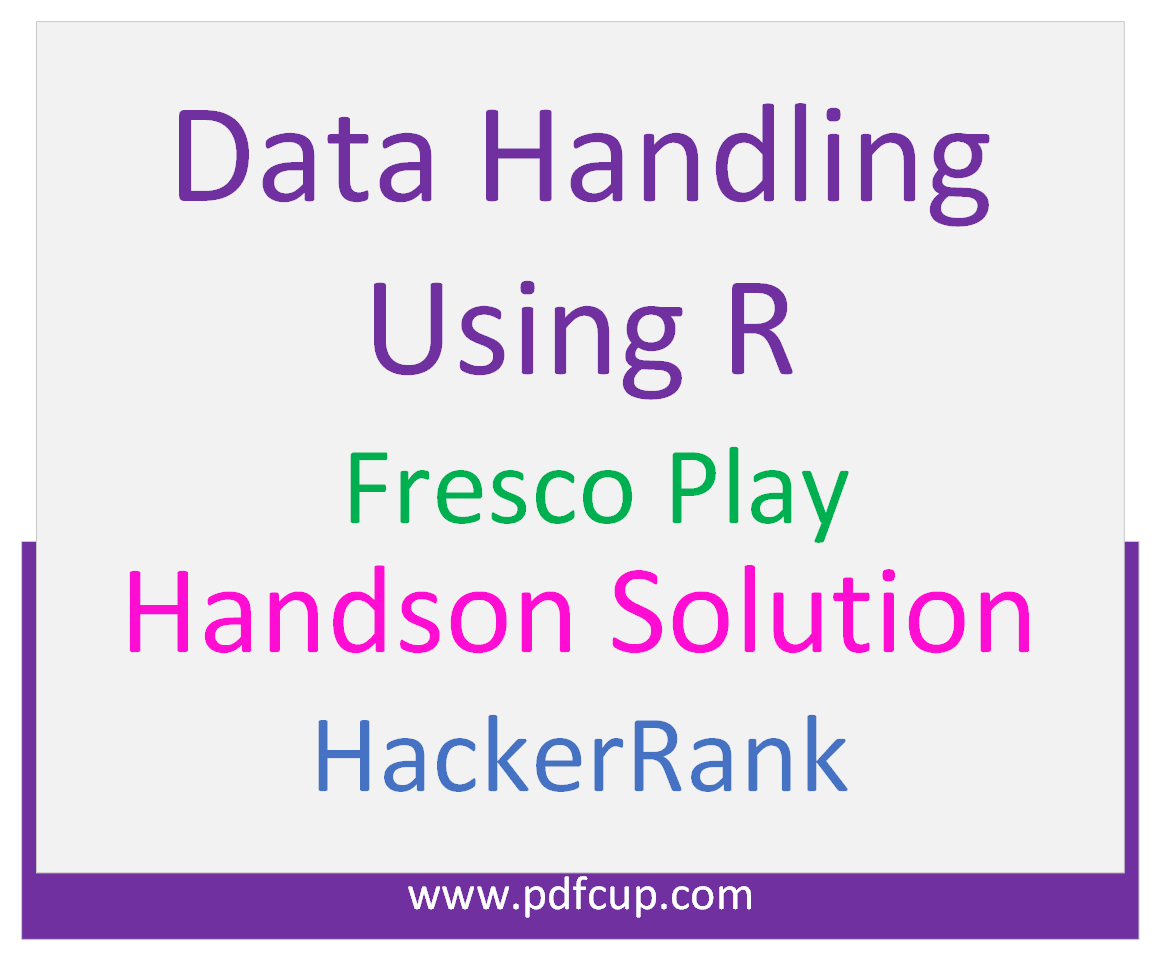
LAB 1: Welcome to Data Handling using R-1 - Data Frames and Datasets in R.
Practice Exercises on Data Frames
Solution: Practice Exercises on Data Frames
data_handle_1 <- function()
{
#Write your code here
# Task 1: Perfrom the following tasks in the function data_handle_1
# A. Create 3 vector (name, age, department) with 5 observations each.
name <- c("A", "B", "C", "D", "E")
department <- c("AA", "BB", "CC" , "DD", "EE")
age <- c(10,11,12,13,14)
# B. Create a data frame empdetails using these three vectors.
empdetails_df <- data.frame(name , age,department )
# C. Print the data frame and view the structure of the data frame and print it.
print(empdetails_df)
print(str(empdetails_df))
#######################
# Task 2: Perform the following tasks:
# A. View the structure in the dataset mtcars and print it.
print(str(mtcars))
# B. Print the summary of the dataset mtcars and print it.
print(summary(mtcars))
#######################
# Task 3: Print the first, second and tenth columns of the R build-in dataset mtcars.
print(mtcars[, c(1, 2,10)])
#######################
# Task 4: Sort and Print mtcars by the column mpg.
print(mtcars[order(mtcars$mpg),])
#######################
#Task 5: From mtcars print details of all cars which provide more than 30 mpg.
task5 <- mtcars[mtcars$mpg > 30, ]
print(task5)
#Task 6: From mtcars and find all cars that provide more than 30 mpg, and print the details of the cars in decreasing order of mileage.
# If there are two rows with same mileage, sort the two rows in decreasing order of disp.
print(task5[rev(order(task5$mpg)),])
}
data_handle_1()
Lab 2: Welcome to Data Handling using R-2 - Data Tables in R
Practice using Data Tables.
Solution:
data_table <- function(){
#Write your code here
library(data.table)
table_df <- as.data.table(mtcars)
filter <- table_df[mpg> 15, .( AvgHp= mean(hp), AvgWt = mean(wt)) , by = list(cyl, carb)]
print(filter)
}
data_table()
Lab 3: Welcome to Data Handling using R-3 - Loop Functions
Practice Exercises on Loop Functions
Solution:
loops <- function(){
#Write your code here
#############################
# Task 1: Consider a class of 5 students,
# A. Create 3 vectors which contians marks scored by the students in the following:
# Maths(maths)- (70,75,80,85,90),
# Science(science) - (71,76,81,86,91),
# English(english)- (73,78,83,88,93).
maths <- c(70,75,80,85,90)
science <- c(71,76,81,86,91)
english <- c(73,78,83,88,93)
# B. Create a list using these 3 Vectors.
student_list <- list(maths, science, english)
# C. Calculate the class average in each of these subjects by using lapply() and print it.
ans1 <- lapply(student_list, mean)
print(ans1)
#############################
# Task 2: Consider a class of 4 students:
# A. Create 4 vector which contain the marks of 4 students in 3 subjects - Maths, Science, and English.
# The values are s1- (70,80,90), s2- (71,81,91), s3 <- c(72,82,92) and s4 <- c(73,83,93).
s1 <- c(70,80,90)
s2 <- c(71,81,91)
s3 <- c(72,82,92)
s4 <- c(73,83,93)
# B. Create a list using the 4 vector.
student_list2 = list(s1,s2,s3,s4)
# C. Calculate the total score(mean) for each of the 4 students using the function sapply() and print it.
total_score <- sapply(student_list2 , mean)
print(total_score)
#############################
# Task 3: Perform the following tasks:
# A. Create a 10X5 Matrix using the numbers from 1 to 50. (Arrange 1 to 50 not in row-wise order.)
# Hint: GIve byrow =FALSE
mtrix <- matrix(c(1:50), nrow = 10, ncol = 5, byrow = FALSE)
# print(mtrix)
# B. Find the Average of each column of the matrix by using the apply() function and print it.
avg_cl <- apply(mtrix, 2, mean)
print(avg_cl)
#############################
# Task 4: For this excerise, let us the the R built-in Dataset mtcars.
# A. Using thr tapply() function, find the average 'mpg' for the various transmission types(am) and number of cylinder(cyl) in a car and print it.
avg_mpg <- tapply( mtcars$mpg, list( mtcars$cyl ,mtcars$am) , mean )
print(avg_mpg)
#############################
# Task 5 : Perform the Following tasks:
# A. Create a vector x with the elements from 1 to 5,
# B. Create another vector y with elements from 6 to 10,
c <- c(1:5)
p <- c(6:10)
# C.Use mapply() to multiply the vector x and y, such that the first element of x is multiplied with the first element of y and print it.
mult_one <- function(Data1,Data2)
{
Data1*Data2
}
cc <- mapply(mult_one,c, p )
print(cc)
}
loops()
LAB 4: Welcome to Data Handling Using R-4 - Introduction to dplyr
Introduction to dplyr
Solution:
library(dplyr, warn.conflicts = FALSE)
dplyrs <- function(){
# print(getwd())
# print(list.files())
# Task 1:
# Include libraries dplyr and read hflights dataset from
# the csv file hflights.csv and save it as data frame in
# the variable name hflights. Use the dim function of
# the dplyr package to discover the dimensionality of
# the dataset. Running this function on a dataset will
# return the number of records and columns in the
# dataset and print it.
hflights <- read.csv("hflights.csv")
print(dim(hflights))
# Task 2.
# Use the functions head () and tail () to take a look
# at some instances of the data. Try running this
# function on the hflights dataset and print it
# separately.
print(head(hflights))
print(tail(hflights))
# Task 3:
# Running the head function on a local data frame,
# prints the given number of rows of the data frame. Try
# running this function on the hflights dataset to print
# 20 records.
print(head(hflights, 20 ))
# Task 4:
# glimpse () is like a transposed version of print.
# Columns run down the page, and data runs across.
# This makes it possible to view every column in a data
# frame.
# Run this function on the hflights dataset. Note - Here
# don't print instead run only glimpse of the dataset.
glimpse(hflights)
# Task 5:
# Perform the following tasks:
# A. Create a data frame hflights1 which will have first fifty rows of the data set hflights
d <- hflights[c(1:50),]
# B. Convert hflights1 into a tbl.
hflights1 <- as_tibble(d)
# C. To see how tbl behaves like data frames, save the UniqueCarrier column of hflights tbl
# as an object named carriers, by using standard R syntax and print it.
carriers <- hflights1$UniqueCarrier
print(carriers)
# Task 6:
# Perform the following tasks:
# A. Create a list abrCarrier which will contain actual carrier names corresponding to the values in the
# variable UniqueCarrier.
abrCarrier < - c("AA" = "American", "AS" = "Alaska", "B6" = "JetBlue", "CO" = "Continental", "DL" = "Delta", "OO" = "SkyWest", "UA" = "United", "US" = "US_Airways", "WN" = "Southwest", "EV" = "Atlantic_Southeast", "F9" = "Frontier", "FL" = "AirTran", "MQ" = "American_Eagle", "XE" = "ExpressJet", "YV" = "Mesa")
# B. Add a new column Carrier to hflights which will contain the actual Carrier name by referring abrCarrier and the UniqueCarrier column of hflights.
hflights <- transform( hflights, Carrier= ifelse(hflights$UniqueCarrier %in% names(abrCarrier), abrCarrier[hflights$UniqueCarrier ], "Error"))
# C. Print the first 10 rows of the data set to view the values in the newly added column.
print(head(hflights, 10))
}
dplyrs()
LAB 5: Welcome to Data Handling Using R-5 - dplyr Verbs and Functions
dplyr Verbs and Functions
Solution: dplyr Verbs and Functions.
library(dplyr, warn.conflicts = FALSE)
dplyr_verbs <- function (){
#Write your code here
hflights <- read.csv("hflights.csv")
# Task 1:
#Include dplyr and read hflights dataset from the csv file hflights.csv and save it as data frame in the variable name hflights.
#1. Find the list of flights in the Data Set hflights with flight number (FlightNum) 428 or 460 and print it. Hint: Use "|"
# Filter the dataset for FlightNum 428 or 460
filtered_flights <- hflights %>%
filter(FlightNum == 428 | FlightNum == 460)
# Print the filtered flights
print(filtered_flights)
# Task 2: Create a data frame hflights1 which will have first twenty rows of the data set hflights.
# While selecting columns, clauses like contains, starts_with, ends_with and matches can be used to match columns by name.
#Challenge: In the hflights, select FlightNum and all columns with names that contain Time and print it.
# Create a data frame with the first twenty rows
hflights1 <- hflights %>%
slice_head(n = 20)
# Select FlightNum and all columns that contain "Time" in their names
hflights1_filtered <- hflights1 %>%
select(FlightNum, contains("Time"))
# Print the resulting data frame
print(hflights1_filtered)
# Task 3:
# In the hflights1 dataset, using the columns, Distance and AirTime, calculate the speed in Km/Hr and print it.
# Calculate speed in Km/Hr
hflights1_speed <- hflights1 %>%
mutate(velocity = (Distance ) / (AirTime / 60))
# Print the resulting data frame with the new Speed_Km_Hr column
print(hflights1_speed)
# Task 4:
#.List all records in the dataset hflights1, sorted by delay in arrival of flight, in descending order. Hint: Use desc(ColumnName) to order a column in descending order and print it.
# newdata <- hflights1[order(hflights1$ArrTime, decreasing = TRUE),]
hflights1_sorted <- hflights1 %>%
arrange(desc(ArrDelay))
# Print the sorted dataset
print(hflights1_sorted)
# Task 5: Find the number of flights being cancelled based on column UniqueCarrier as per the Data Set hflights and print it.
cancelled_flights_by_carrier <- hflights %>%
group_by(UniqueCarrier) %>% # Group by UniqueCarrier
summarize(n_flights = n(), n_canc = sum(Cancelled) ) # Count the number of canceled flights
# Print the results
print(cancelled_flights_by_carrier)
# Task 6: Using the summarise and group_byfunctions on the dataset hflights,
# find out the number of flights that flew from Houston by Month, and the average delay in arrival and print it.
# Find the number of flights from Houston by month and average delay
summary_flights <- hflights %>%
filter(Origin == "IAH") %>% # Filter flights originating from Houston (IAH)
group_by(Month) %>% # Group by month
summarize(
mean(ArrDelay, na.rm = TRUE) # Calculate the average arrival delay
)
# Print the results
print(summary_flights)
}
dplyr_verbs()
LAB 6: Welcome to Data Handling Using R-6 - Set Operations in dplyr
Set Operations in dplyr
Solution:
sets <- function() {
library(dplyr)
#Write your code here
# Perform the following tasks in the function sets
# 1.mtcars is an existing dataset in R.
# * Create two vectors first and second with each containing rows 1 to 6, and rows 6 to 15 respectively.
# These two data frames will be used to perform set operations.
# Task 1:
first <- mtcars[c(1:6),]
row.names(first) <- NULL
second <- mtcars[c(6:15),]
row.names(second) <- NULL
# Task 2: Perform the following tasks:
# Run the set operations 'intersect()', union(),union_all() and setdiff() on the objects/data frames first and second and print it.
print(intersect(first, second))
print(union(first, second))
print(union_all(first, second))
print(setdiff(first, second))
}
sets()
LAB 7: Welcome to Data Handling Using R-7 - Contingency Tables
Pactice Creating Contingency Tables
Solution:
#Write your code here
# Task1: Apply the function 'table, cut and preety' on the R build-in Data Set 'mtcars'.
# And create a contingency table based on mpg provided by the listed cars and print it.
break_point = pretty(mtcars$mpg)
column_vector = mtcars$mpg
result <- table(cut(column_vector, break_point))
print(result)
LAB 8: Welcome to Data Handling Using R - Test Your Knowledge
Test Your Knowledge
Solution:
#Note: If you faced any issue while compiling the code, kindly discuss the issue on the comment box.
# Enter your code here. Read input from STDIN. Print output to STDOUT.
library(dplyr)
data_oper <- function(){
Try to solve it by yourself.
}
data_oper()